Financial Forecasting for Value Stream-Based Startups
In the exhilarating journey of scaling a startup, few capabilities separate market leaders from the pack more definitively than strategic financial forecasting aligned with value streams. While many founders view forecasting as a necessary evil—an exercise in spreadsheet manipulation to satisfy investors—the most successful scaleups approach it differently. For them, financial forecasting isn't about perfectly predicting an unknowable future. It's about creating a strategic framework that guides decision-making across value streams, identifies risks before they materialize, and illuminates opportunities others might miss.
"Most startups treat financial forecasting like weather prediction—hoping to guess exactly what will happen," explains Maria Lemos, former CFO of a fintech unicorn. "But that misses the point entirely. The real value lies in understanding the range of possible outcomes for each value stream and what drives them. This insight enables you to make better decisions today, regardless of which future unfolds."
This strategic perspective on financial forecasting connects directly to the "F" in our FUND framework—the foundational element of financial strategy for scaling startups that we've explored in our master article. Financial forecasting isn't just about producing numbers; it's about building an evolving model of your business organized around value streams that becomes increasingly powerful as you scale.
For early-stage startups running on instinct and energy, basic financial projections might suffice. But as you scale—adding customers, employees, and complexity—robust value stream-based forecasting capabilities become critical. Market conditions shift rapidly. Cash runways shorten unexpectedly. Growth opportunities emerge suddenly. Without sophisticated forecasting practices organized around how value flows to customers, scaling companies find themselves making crucial decisions based on gut feeling rather than insight, often with costly consequences.
In this article, we'll explore how to transform financial forecasting from a compliance exercise into a strategic advantage for your scaling, value stream-based startup. We'll examine the evolution of forecasting capabilities across growth stages, dive into practical implementation techniques organized around value delivery, and showcase real-world examples of companies that have used value stream financial forecasting to navigate the challenges of rapid growth. Whether you're preparing for your next funding round or building systems to support sustainably accelerating growth, these practices will help you create forecasting capabilities that illuminate rather than obscure your path forward.
Let's dive into how financial forecasting can become your strategic compass in the often turbulent waters of scaling a value stream-based startup.
The Evolution of Financial Forecasting in Value Stream-Based Startups
Financial forecasting capabilities must evolve as your company grows, particularly as you organize around value streams rather than traditional functional departments. The approaches that work for a pre-revenue startup will quickly prove insufficient for a company scaling across markets with multiple value streams and complex customer journeys.
Early Stage: Proving the Model (Pre-Series A)
At the earliest stages, financial forecasting focuses primarily on runway and basic viability, with emerging attention to key value streams:
Focus Areas: Cash runway is the primary concern, with detailed tracking of burn rate and cash reserves. Startups at this stage typically maintain rolling 12-18 month cash forecasts, updated monthly to reflect changing conditions. While revenue projections exist, they're often aspirational rather than predictive. Early value stream identification begins to emerge, focusing on the core paths through which value is delivered to customers.
Typical Approaches: Simple spreadsheet models suffice at this stage, usually maintained by founders or fractional CFOs. The models generally use high-level assumptions about market size, penetration rates, and conversion funnels. Monthly review cadences are common, with particular focus on variances in cash burn rather than revenue prediction accuracy. Value streams tend to be broadly defined, often simply as "customer acquisition" and "product delivery."
Common Challenges: The most significant forecasting challenge at this stage is balancing optimism with reality while beginning to identify key value streams. Early-stage founders necessarily believe in ambitious growth trajectories—without this optimism, they wouldn't be entrepreneurs—but overly rosy projections can lead to premature scaling and dangerous cash shortfalls. Simultaneously, they must begin mapping how value flows to customers to create the foundation for more sophisticated forecasting as they scale.
A B2B software startup I advised hit this challenge directly. Their initial forecasts showed profitability within 12 months, but a more rigorous analysis revealed this required conversion rates three times industry averages. We created a revised forecast with three scenarios, helping them make more realistic hiring and investment decisions that extended their runway through a critical product development phase. Simultaneously, we mapped their two core value streams—customer onboarding and service delivery—to better understand the drivers of customer success.
Growth Stage: Building Scale Around Value Streams (Series A to B)
As companies establish product-market fit and begin scaling rapidly, forecasting becomes more sophisticated and organized explicitly around value streams:
Focus Areas: While cash remains crucial, forecasting now extends to operational metrics like customer acquisition costs, conversion rates, and lifetime value for each value stream. Growth-stage companies typically implement cohort-based revenue forecasting to understand how different customer segments behave over time within each value stream. Expense forecasting becomes more detailed, with growing attention to unit economics and efficiency metrics by value stream.
Typical Approaches: Driver-based forecasting emerges at this stage, linking key business drivers (like conversion rates or churn) directly to financial outcomes within each value stream. Simple statistical models begin appearing, using regression analysis to identify trends and seasonality. Quarterly forecasting cycles become common, with weekly or biweekly variance analysis to catch emerging trends early. Value streams are more clearly defined, typically covering the full customer journey from acquisition through expansion and retention.
Common Challenges: The primary forecasting challenge for growth-stage, value stream-based companies is managing rapid change across multiple value flows. When you're growing 10-30% monthly, historical patterns quickly become obsolete. The best forecasting approaches at this stage incorporate rapid feedback loops, continuously updating assumptions based on the latest data for each value stream. This approach requires building more sophisticated data infrastructure and dedicating resources specifically to financial analysis organized around value delivery.
A direct-to-consumer company I worked with addressed this challenge by implementing weekly forecasting updates focused on their critical metrics within each value stream: CAC by channel (acquisition stream), conversion rates (onboarding stream), and repeat purchase patterns (retention stream). This cadence allowed them to identify a significant shift in Facebook acquisition costs two weeks before it would have shown up in their monthly forecasts, enabling quick budget reallocation that maintained their growth trajectory.
Scale Stage: Value Stream Excellence (Series B and Beyond)
As companies mature further, financial forecasting becomes deeply integrated with strategic planning and operational execution across an expanded set of value streams:
Focus Areas: Forecasting at this stage encompasses comprehensive business planning, with detailed revenue projections by product, channel, and geography for each value stream. Scenario planning becomes sophisticated, with explicit modeling of various market conditions and competitive responses for each value stream. Forecasting extends to balance sheet and cash flow projections, not just P&L, with growing attention to capital efficiency and investor metrics. Value streams may be nested or interconnected, with clear understanding of how they support each other.
Typical Approaches: Advanced statistical methods and potentially machine learning models emerge, integrating both internal data and external market signals for each value stream. Rolling forecasts become standard, typically looking 18-24 months forward with decreasing detail for outer periods. Dedicated financial planning and analysis (FP&A) teams manage the forecasting process, working closely with value stream leaders across the business. Value stream mapping becomes sophisticated, capturing complex interdependencies.
Common Challenges: The greatest forecasting challenge for scaling, value stream-based companies is maintaining agility while managing complexity across multiple value streams. As operations grow across products and markets, forecasting models risk becoming unwieldy and divorced from operational reality. The most successful companies implement forecasting technologies and processes that balance comprehensiveness with usability, ensuring forecasts remain valuable strategic tools rather than compliance exercises.
A fintech platform I advised tackled this by implementing a driver-based forecasting system organized around their five core value streams (user acquisition, onboarding, transaction processing, lending, and customer growth). Their system automatically ingested data from their core systems but maintained focus on the 12-15 key metrics that truly drove each value stream. This approach provided comprehensive coverage while keeping their models understandable and actionable for their executive team.
Building Your Value Stream-Based Forecasting Capability
Now that we understand how financial forecasting evolves in value stream-based organizations, let's examine how to implement effective forecasting practices within your organization. Rather than focusing narrowly on particular forecasting techniques, we'll explore the broader capabilities needed to make forecasting a strategic asset aligned with value delivery.
Implementing Value Stream-Based Driver Forecasting
Traditional forecasting often relies on simple growth percentages applied to historical results at a departmental level. This approach fails to capture the complex dynamics of scaling businesses organized around value streams. Value stream-based driver forecasting provides a more powerful alternative:
Mapping Your Value Streams
The foundation of effective forecasting in a value stream-based organization is a clear map of how value flows to customers:
- Identify the core value streams in your business (typically 3-7 major streams)
- Document how these streams deliver value to customers
- Map the key activities, resources, and interdependencies within each stream
- Define the boundaries and interfaces between streams
A healthcare technology company I advised identified four primary value streams: provider acquisition, patient onboarding, care delivery, and health management. By mapping these streams clearly, they created the foundation for more insightful forecasting that directly connected to customer outcomes.
Identifying Key Value Stream Drivers
With value streams clearly mapped, the next step is identifying the vital few metrics that drive performance within each stream:
- For acquisition streams: Lead generation efficiency, conversion rates, sales cycle length
- For onboarding streams: Setup time, activation rates, initial usage metrics
- For delivery streams: Fulfillment speed, service quality, resource utilization
- For retention streams: Engagement frequency, expansion rates, churn signals
The key is focusing on the "input metrics" that ultimately drive financial outcomes rather than the outcomes themselves. A marketplace startup I worked with identified three key drivers for each of their value streams: supply partner acquisition cost, demand conversion rate, and transaction frequency. By understanding these drivers deeply for each stream, they built far more insightful forecasts than by simply projecting historical trends.
Building Value Stream Driver Models
Once you've identified your key drivers for each value stream, the next step is creating mathematical relationships between these drivers and your financial results:
- Gather historical data on both drivers and outcomes for each value stream
- Analyze the relationships between them (using tools ranging from simple correlation analysis to more sophisticated statistical methods)
- Create formulas that translate driver inputs into financial outputs by value stream
- Test these formulas against historical data to validate their accuracy
A B2B software company built a driver model for each of their value streams that linked marketing spend by channel to pipeline generation, conversion rates by sales stage, implementation timelines, and ultimately revenue recognition. This model allowed them to test different investment scenarios across value streams and quickly identify the most efficient paths to growth.
Implementing Value Stream Sensitivity Analysis
A powerful aspect of value stream-based driver forecasting is the ability to understand which drivers in which streams have the greatest impact on your results. Sensitivity analysis involves systematically varying each driver to see how it affects outcomes. This analysis often reveals non-obvious insights about your business model.
For example, a healthcare technology company discovered through sensitivity analysis that a 10% improvement in user activation in their onboarding value stream had four times the impact on lifetime value as a 10% improvement in customer acquisition cost in their acquisition stream. This insight led them to reallocate resources from marketing optimization to user onboarding, significantly improving their unit economics.
Creating Robust Financial Scenarios Across Value Streams
Single-point forecasts create a false sense of precision in an inherently uncertain environment. Value stream-based scenario planning provides a more honest and useful approach to navigating the future:
Defining Your Value Stream Scenario Framework
Rather than creating arbitrary "best case" and "worst case" scenarios, effective scenario planning is built around key uncertainties specific to each of your value streams:
- Identify the critical uncertainties that could significantly impact each value stream (e.g., competitive actions, regulatory changes, market adoption rates)
- For each uncertainty, define plausible alternative outcomes
- Create coherent scenarios by combining these outcomes in logical ways across value streams
- Develop detailed financial projections for each scenario, showing impacts on each value stream
A fintech company I advised built their scenarios around two critical uncertainties for each of their core value streams. For their acquisition stream, they focused on regulatory change and customer acquisition costs. For their lending stream, they examined interest rate scenarios and default rates. By examining these combinations, they identified strategies that would work well regardless of which future emerged.
Building Value Stream Contingency Triggers
The real power of scenario planning comes from linking it directly to decision-making through contingency triggers—predefined conditions for specific value streams that automatically activate specific action plans:
- Leading indicators for each value stream that signal which scenario is emerging
- Specific thresholds that warrant action within each stream
- Predefined response plans for each threshold crossing
A direct-to-consumer startup implemented contingency triggers for their acquisition value stream centered around their customer acquisition costs. If CAC increased more than 20% above forecast for two consecutive weeks, they automatically shifted budget to their most efficient channels and accelerated the launch of retention-focused features in their engagement value stream. This approach allowed them to respond to market changes within days rather than weeks.
Communicating Value Stream Scenarios Effectively
Scenarios are only valuable if they inform decision-making throughout the organization. Effective communication approaches include:
- Executive dashboards showing current status for each value stream relative to scenario boundaries
- Regular scenario review sessions discussing emerging signals and trends by value stream
- Clear visualization of scenario implications for key metrics across value streams
A B2B software company created a simplified scenario dashboard for their board and leadership team, showing their current trajectory for each value stream relative to their four core scenarios. This visualization made complex uncertainties discussable and actionable, improving strategic alignment across the organization.
Implementing Rolling Forecasts Across Value Streams
Traditional annual forecasting cycles struggle to keep pace with the rapidly changing conditions of scaling, value stream-based startups. Rolling forecasts organized by value stream provide a more adaptive alternative:
Designing Your Value Stream Rolling Forecast Process
Rolling forecasts maintain a consistent time horizon (typically 4-6 quarters) that moves forward each month or quarter, with clear visibility into each value stream. Implementing this approach involves:
- Defining your rolling time horizon for each value stream (how far forward you'll forecast)
- Establishing your update frequency (monthly or quarterly) by value stream
- Determining the level of detail for near-term vs. longer-term periods within each stream
- Creating a streamlined process for regular updates
A healthcare technology company implemented a 6-quarter rolling forecast updated monthly for each of their four value streams. For each stream, the first quarter was detailed by week, the second quarter by month, and remaining quarters showed monthly totals. This approach provided granular visibility where it mattered most while maintaining a strategic view further out.
Balancing Thoroughness with Efficiency Across Value Streams
The greatest challenge with rolling forecasts is managing the workload of frequent updates across multiple value streams. Successful implementations:
- Automate data collection for each value stream wherever possible
- Focus detailed analysis on significant variances rather than every line item
- Use driver-based approaches to simplify updates across streams
- Implement collaborative forecasting tools rather than relying on email and spreadsheets
A marketing technology company automated 80% of their forecast data collection by integrating their CRM, marketing automation, and financial systems. This automation allowed their financial team to focus on analysis rather than data gathering, significantly improving both the quality and efficiency of their forecasting process across value streams.
Creating Accountability Through Value Stream Forecast Accuracy Metrics
Rolling forecasts improve over time when value stream teams take ownership of their accuracy. Implementing forecast accuracy metrics by value stream helps create this ownership:
- Track forecast vs. actual variances over time for each value stream
- Analyze patterns in these variances by stream (e.g., consistent over-forecasting)
- Create friendly competition around forecast accuracy between value streams
- Celebrate improvements in forecasting capability
A B2B software company implemented quarterly awards for the most accurate value stream forecasts. This simple gamification dramatically improved forecast quality as value stream leaders invested more time understanding their drivers and trends. Importantly, they celebrated honest forecasts rather than sandbagged ones, creating a culture of transparency across value streams.
Making Value Stream Financial Forecasting a Strategic Asset in Your Startup
Building effective forecasting capabilities around value streams isn't primarily about adopting specific techniques—it's about creating the right organizational approach to forecasting. Here are key principles for transforming forecasting into a strategic asset:
Foster a Culture of Value Stream Forecast Integrity
The foundation of effective forecasting is organizational honesty—the willingness to confront reality rather than wishful thinking. Creating this culture involves several elements:
Separate Value Stream Targets from Forecasts
Many forecasting problems stem from conflating targets (what you want to happen) with forecasts (what you expect to happen) for each value stream. These serve different purposes and should be explicitly separated in your planning processes. Targets should be ambitious and motivating; forecasts should be realistic and planning-oriented.
A B2B software company I advised implemented a two-column approach in their value stream planning documents: one column for targets and another for forecasts. This simple visual separation clarified thinking throughout the organization and led to more honest conversations about performance gaps within each stream.
Celebrate Value Stream Forecast Accuracy, Not Just Performance
The behaviors you recognize shape your culture. When you celebrate accurate forecasts for each value stream regardless of whether the news is good or bad, you encourage the transparency needed for effective planning. Conversely, when you punish bearers of bad news, you guarantee optimistic forecasts disconnected from reality.
A healthcare technology company implemented quarterly "crystal ball awards" for the most accurate value stream forecasts. Importantly, the award criteria explicitly excluded sandbagging (deliberately low forecasts), focusing instead on genuine predictive accuracy. This simple recognition significantly improved forecast quality over time across all value streams.
Create Safety for Transparent Value Stream Communication
Team members will only provide honest forecasts when they feel psychologically safe doing so. Creating this safety requires consistent leadership behavior, particularly when handling negative variances or missed forecasts within value streams.
A fintech startup implemented a "no surprise" policy—value stream leaders could bring any forecast variance to leadership without fear, but hiding or delaying bad news was unacceptable. This approach dramatically improved information flow throughout the organization, enabling faster course corrections when challenges emerged in any value stream.
Implement the Right Value Stream Forecasting Technology
As you scale, spreadsheet-based forecasting becomes increasingly problematic—creating version control issues, calculation errors, and collaboration challenges. Implementing appropriate technology significantly enhances forecasting capabilities:
Evaluate Value Stream-Friendly Forecasting Solutions
Numerous technologies now exist specifically for financial planning and forecasting. The best tools for value stream-based organizations provide:
- Flexible hierarchical structures that can model value streams and their components
- Driver-based modeling capabilities that connect operational metrics to financial outcomes
- Collaborative features that enable input from distributed value stream leaders
- Scenario planning capabilities to test different assumptions for each value stream
A B2B software company transitioning from spreadsheet-based planning to a dedicated value stream forecasting platform found they reduced their forecasting cycle time by 60% while simultaneously increasing the sophistication of their analysis. This improved both the timeliness and quality of their business decisions across value streams.
Integrate Your Value Stream Data Ecosystem
Forecasting power increases dramatically when your forecasting system integrates with the operational data sources for each value stream. Key integrations typically include:
- CRM systems for acquisition value stream data
- Product analytics for usage and engagement value stream metrics
- Customer service platforms for support value stream metrics
- ERP or accounting systems for financial results across all streams
A direct-to-consumer company implemented APIs that automatically pulled data from their e-commerce platform, marketing tools, and inventory system into their value stream forecasting solution. This integration eliminated manual data entry errors and allowed them to update forecasts weekly rather than monthly, significantly improving their reaction time to changing market conditions.
Implement Collaborative Value Stream Workflows
Effective forecasting requires input from value stream leaders across the organization. Modern forecasting tools support collaborative workflows that:
- Allow distributed input from value stream leaders
- Track changes and comments for accountability
- Implement approval workflows for forecast updates
- Enable scenario testing and sensitivity analysis by value stream
A fintech platform implemented a collaborative value stream forecasting solution that allowed each value stream leader to input their own forecasts through a guided workflow. This distributed approach improved ownership, accuracy, and cross-functional alignment while reducing the finance team's administrative burden.
Build Your Value Stream Forecasting Roadmap
Forecasting capabilities evolve over time. Rather than attempting a comprehensive transformation overnight, implement a phased approach that delivers incremental value:
Phase 1: Value Stream Foundation (1-3 Months)
Focus on establishing basic value stream forecasting infrastructure:
- Map key value streams and their boundaries
- Identify key drivers for each value stream
- Build simplified driver-based models for each stream
- Implement monthly value stream reviews with structured variance analysis
Phase 2: Value Stream Enhancement (3-6 Months)
Deepen your forecasting capabilities within and across value streams:
- Expand driver-based modeling to all aspects of each value stream
- Implement rolling forecasts with regular update cycles
- Develop cohort-based analysis for customer metrics within relevant streams
- Create value stream-specific scenario planning with explicit linkage to strategic decisions
Phase 3: Value Stream Sophistication (6-12 Months)
Add advanced capabilities that provide deeper insight across your value stream network:
- Implement probabilistic forecasting techniques for each value stream
- Develop advanced scenario planning with Monte Carlo simulation
- Build predictive analytics capabilities using statistical methods or machine learning
- Integrate forecasting directly with strategic planning and resource allocation processes
A marketplace startup followed this phased approach, starting with basic driver models for their core value streams. As these models proved valuable, they gradually expanded to full P&L forecasting and eventually sophisticated market-level projections. This incremental approach allowed them to demonstrate value quickly while building towards a comprehensive value stream-based capability.
Conclusion
Financial forecasting in value stream-based scaling startups isn't about predicting the future with perfect accuracy. In rapidly changing markets with emerging business models, such precision is impossible. Instead, effective forecasting is about creating a structured way to think about the future of each value stream—a strategic compass that helps you navigate through uncertainty while maintaining sight of your destination.
The companies that scale most successfully recognize that forecasting isn't merely a financial exercise—it's a strategic capability that permeates their entire organization. They build forecasting approaches organized around value streams that balance sophistication with usability, integrate with their broader data ecosystem, and directly inform their most important decisions. Most importantly, they create forecasting cultures that value transparency and learning over perfect prediction.
As you implement the practices we've explored—value stream mapping, driver-based modeling, scenario planning, rolling forecasts, and technological integration—remember that the goal isn't forecasting perfection. It's building an evolving model of your business organized around how value flows to customers that becomes increasingly powerful as you gather more data and deeper insights. This model becomes a shared language for discussing strategic options, allocating resources across value streams, and navigating the inevitable challenges of scaling.
The journey from basic spreadsheet projections to sophisticated value stream forecasting capabilities takes time and investment. Start with the foundational elements most relevant to your current stage, build capabilities incrementally, and focus relentlessly on extracting practical value rather than theoretical sophistication. As your forecasting capability matures, it will become an increasingly powerful lens through which you can see your value streams more clearly and make better decisions about their future.
In a scaling environment where conditions change rapidly and resources remain constrained, the ability to see around corners and adapt quickly becomes a significant competitive advantage. By building robust value stream-based forecasting capabilities, you create exactly this advantage—a strategic early warning system that helps you identify both risks and opportunities before others notice them. In markets where the spoils often go to the most agile and insightful, this capability isn't just nice to have—it's increasingly essential for sustainable success.
FAQ Section: Financial Forecasting for Scaling Startups
Q1: How often should scaling startups update their financial forecasts?
A1: Most successful scaling startups implement rolling forecasts updated at least monthly, with weekly updates for rapidly changing metrics like customer acquisition costs or conversion rates. The optimal frequency depends on your growth rate and market volatility. Companies growing 10%+ monthly typically benefit from weekly updates to core metrics, while those with longer sales cycles might manage with monthly full-forecast updates and weekly pipeline reviews.
Q2: When should we move beyond spreadsheets for financial forecasting?
A2: Spreadsheets typically become insufficient when: (1) Multiple people need to collaborate on forecasts simultaneously, (2) Your model requires complex relationships between drivers or historical analysis, (3) Data volumes make manual updates time-consuming and error-prone, or (4) Version control becomes problematic. For most startups, this transition point occurs around Series A when dedicated finance leadership joins and forecasting becomes more sophisticated.
Q3: How can we improve the accuracy of our financial forecasting?
A3: Focus on understanding drivers rather than outcomes. Identify the key metrics that truly drive your business (conversion rates, churn, etc.) and build models that connect these drivers to financial results. Implement cohort analysis to understand how customer behavior evolves over time. Regularly analyze forecast vs. actual variances, identifying patterns and root causes. Create a culture that values forecast integrity over optimistic projections.
Q4: Should we forecast by product, geography, customer segment, or some other dimension?
A4: The right forecasting dimensions depend on what drives variability in your business. Forecast separately along any dimension where behavior differs significantly. For example, if enterprise customers have different acquisition costs, conversion rates, and lifetime values than SMB customers, forecast these segments separately. Similarly, if different products or geographies show distinct patterns, break those out individually.
Q5: How can we forecast when we have limited historical data?
A5: With limited data, focus on: (1) Breaking down forecasts into component drivers that can be researched separately (e.g., market size, penetration rates, conversion percentages), (2) Using industry benchmarks and comparables as starting points, clearly marking which assumptions are externally derived, (3) Implementing shorter forecasting cycles with frequent updates as you gather more data, and (4) Using scenario planning to explicitly account for the uncertainty in your projections.
Q6: How should we communicate forecasts to different stakeholders?
A6: Tailor forecast communication to each audience. For boards and investors, focus on key drivers, unit economics, and cash runway across different scenarios. For executives, emphasize operational metrics that drive financial outcomes and highlight decision points. For department leaders, connect forecasts directly to their KPIs and resource needs. Always communicate the key assumptions underlying forecasts and the range of potential outcomes.
Q7: How can we balance optimism with realism in our financial forecasts?
A7: Separate targets (what you aim to achieve) from forecasts (what you expect to happen). Document both, but be clear about which is which. Implement scenario planning that includes optimistic, base, and conservative cases, with clear probability assessments for each. Create a culture that rewards accurate forecasting rather than just hitting targets. Implement forecast accuracy metrics that track performance over time.
Q8: How should we handle forecast variances when they occur?
A8: Treat variances as learning opportunities, not failures. Implement a structured variance analysis process that: (1) Quantifies the variance by category and driver, (2) Identifies root causes rather than symptoms, (3) Distinguishes between one-time events and systemic issues, (4) Updates future forecasts based on insights gained, and (5) Creates action plans for addressing any underlying problems. The goal is learning and improvement, not blame assignment.
Disclaimer
This blog post was initially generated using Inno Venture AI, an advanced artificial intelligence engine designed to support digital product development processes. Our internal team has subsequently reviewed and refined the content to ensure accuracy, relevance, and alignment with our company's expertise.
Inno Venture AI is a cutting-edge AI solution that enhances various aspects of the product development lifecycle, including intelligent assistance, predictive analytics, process optimization, and strategic planning support. It is specifically tailored to work with key methodologies such as ADAPT Methodology® and Scaleup Methodology, making it a valuable tool for startups and established companies alike.
Inno Venture AI is currently in development and will soon be available to the public. It will offer features such as intelligent product dashboards, AI-enhanced road mapping, smart task prioritization, and automated reporting and insights. If you're interested in being among the first to access this powerful AI engine, you can register your interest at https://innoventure.ai/.
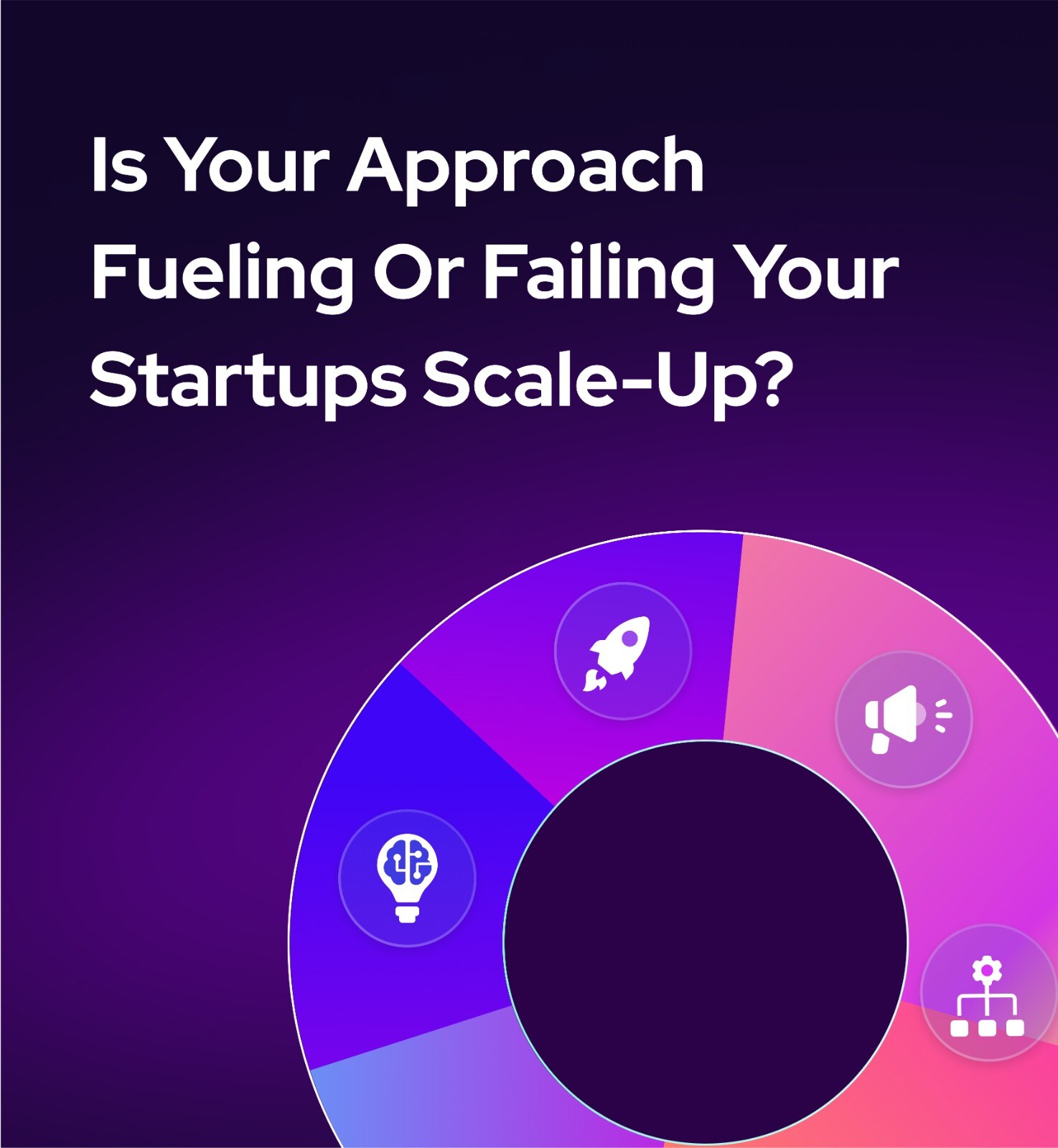
Take The Test
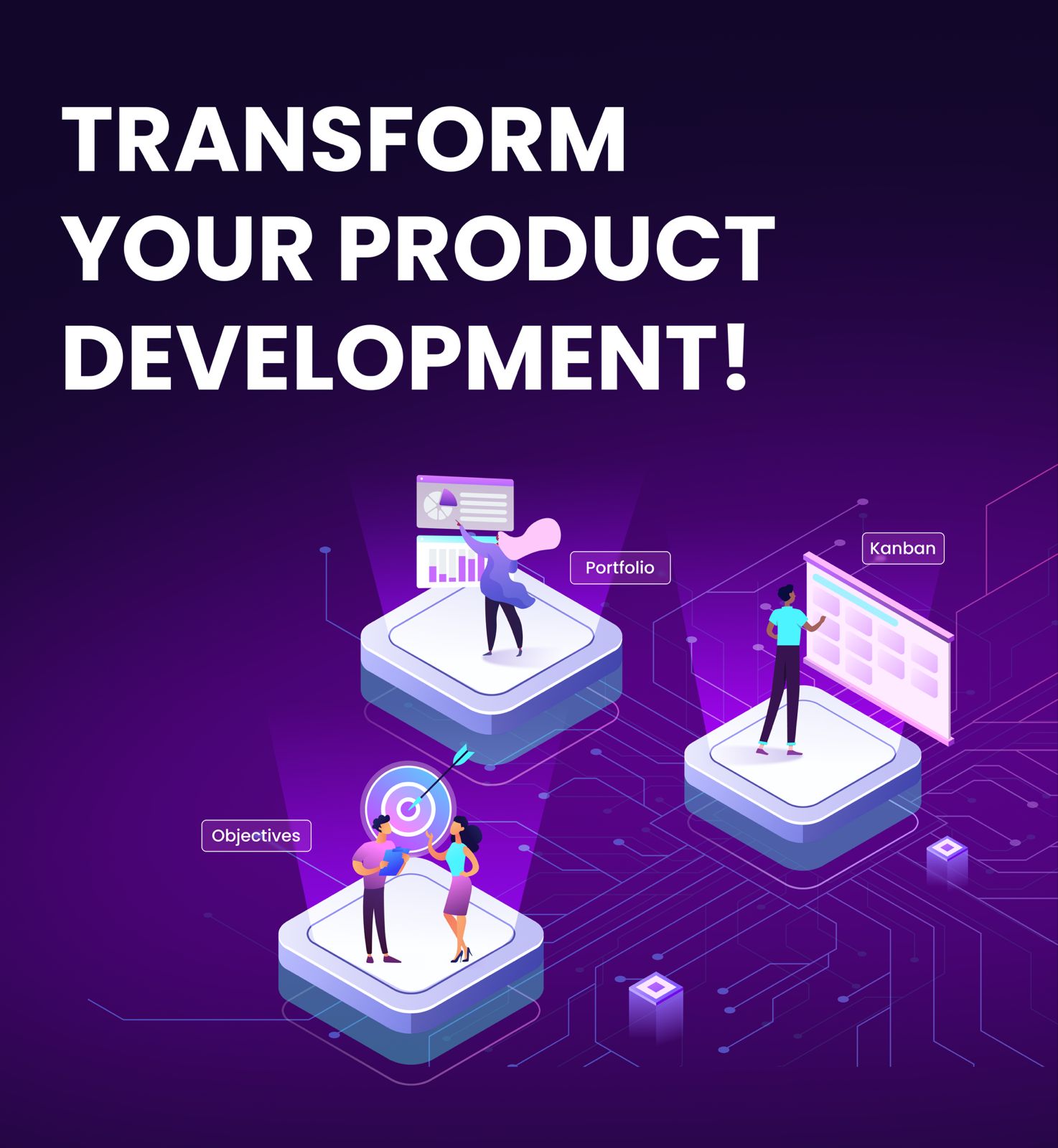
Sign Up
You May Also Like
These Related Stories
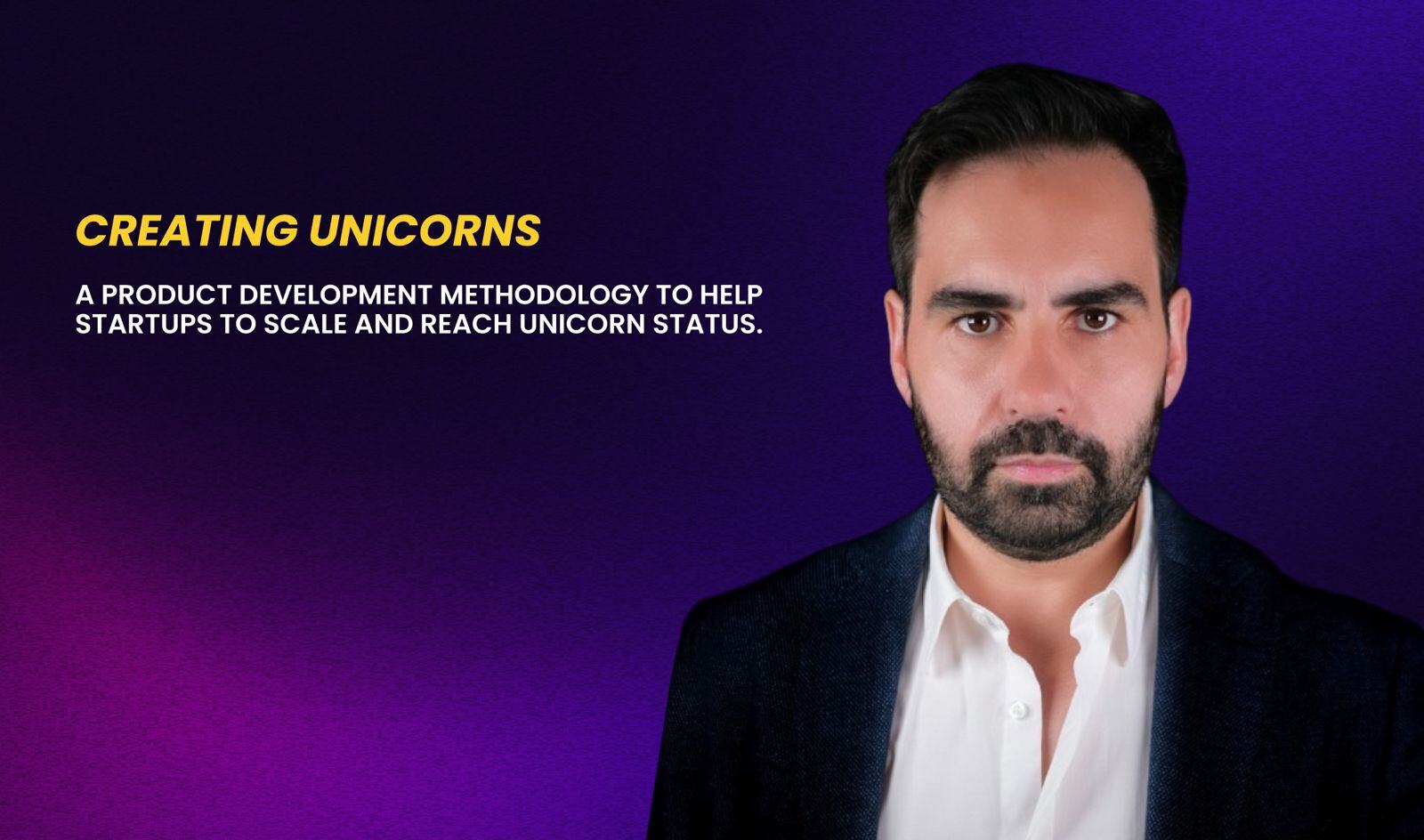
Funding Strategy: Building a Capital Roadmap for Scaling Startups
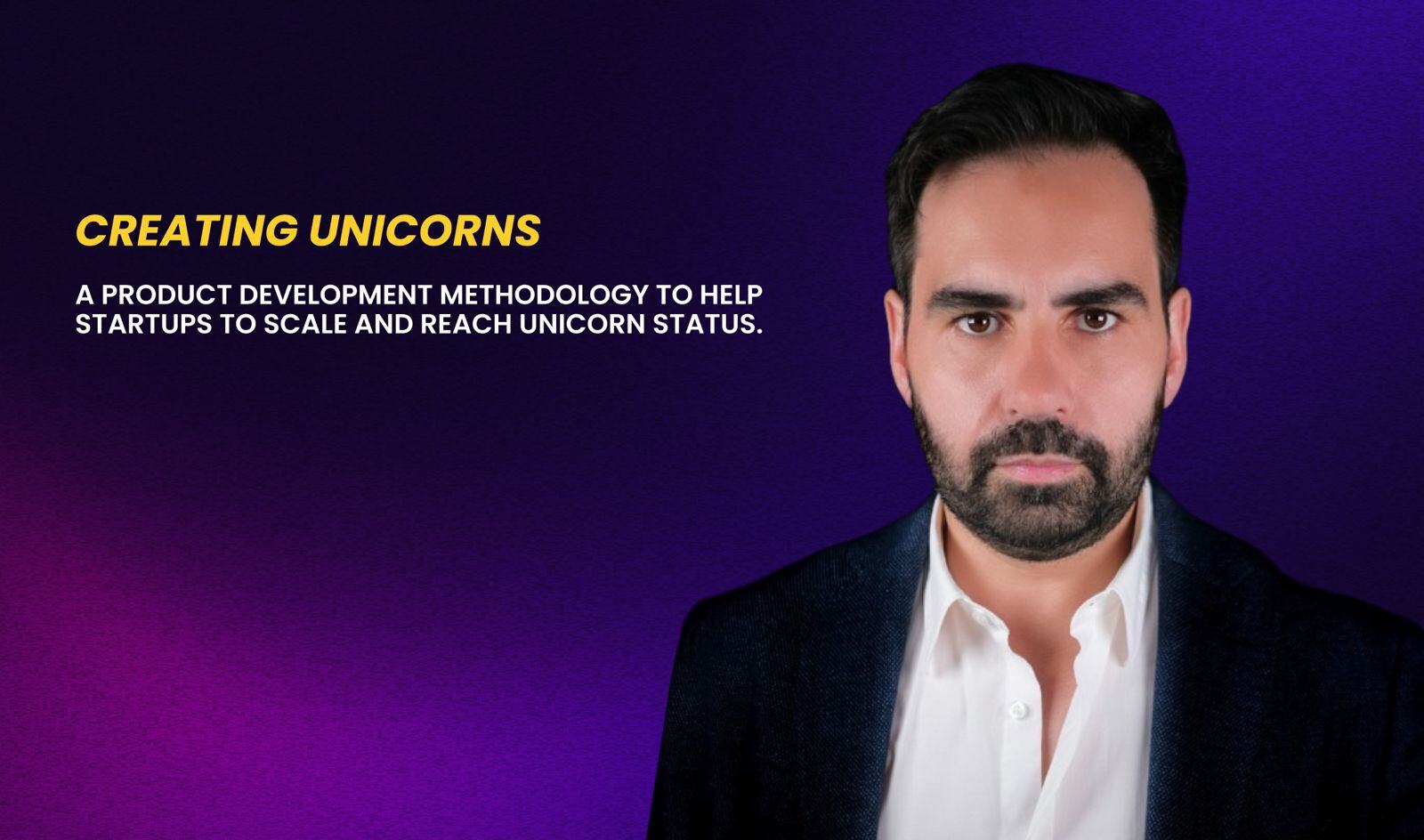
Financial Reporting: A Critical Pillar For Scaling Startups
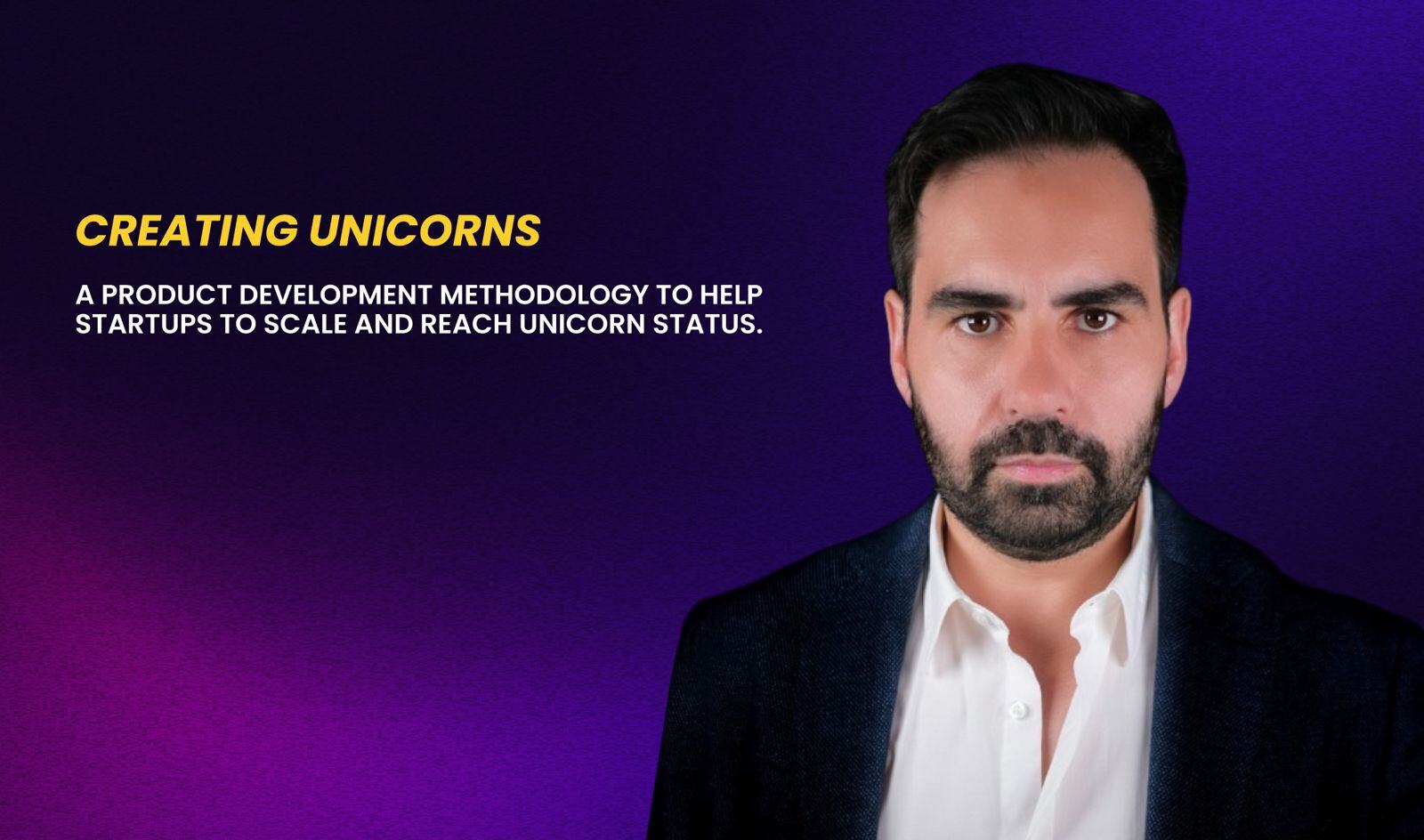